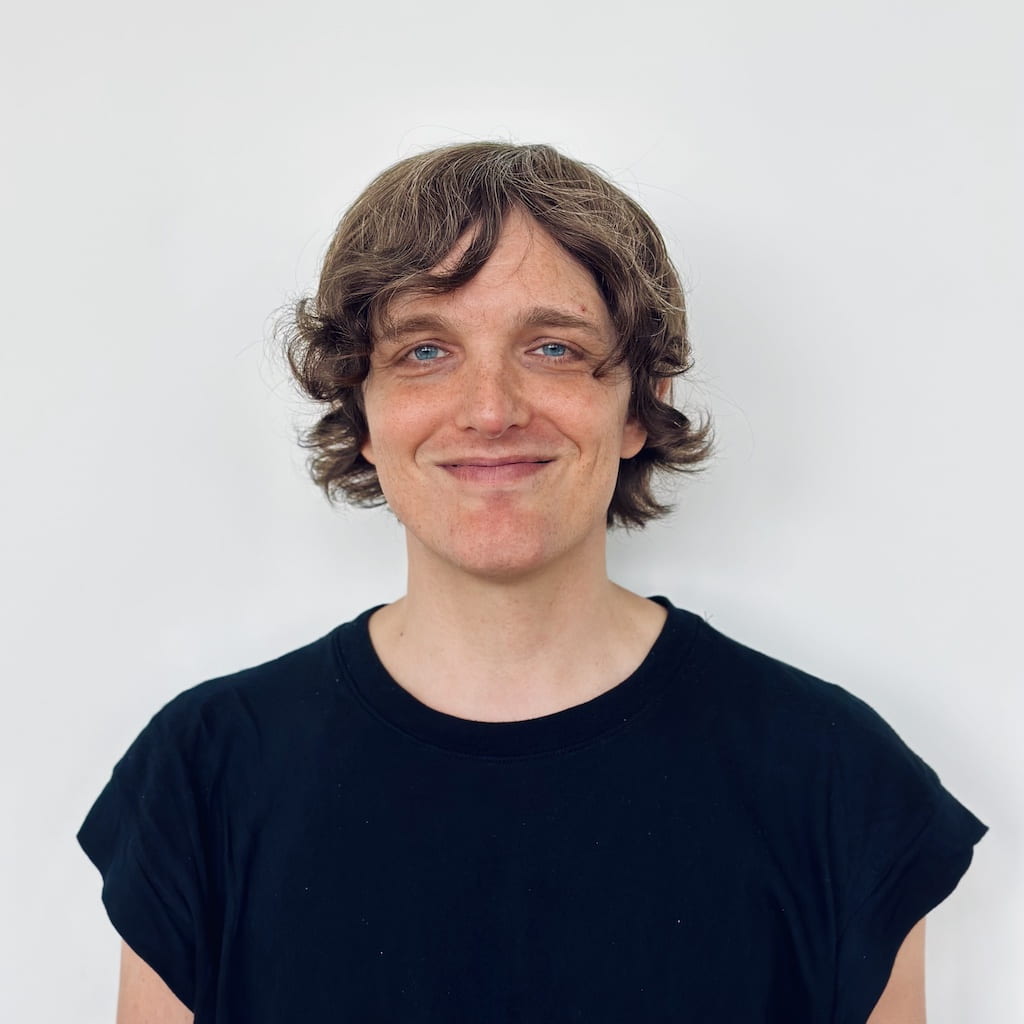
Abstract: Since its emergence roughly 5 years ago, the field of learned data compression has attracted considerable attention. Using machine learning in source coding promises faster innovation cycles, as well as better adaptation to novel data modalities and nonlinear distortion metrics. For example, image codecs can now be end-to-end optimized to perform best for specific types of images, by simply replacing the training set. They may be designed to minimize a given perceptual metric, or in fact any differentiable perceptual loss function, without the need to evaluate it during encoding. In this talk, I will first give an overview of the current state of learned image compression, and then focus on what I consider the next big milestone: finding new and better ways to model visual perception.
Bio: Johannes Ballé (they/them) is a Staff Research Scientist at Google. They defended their master’s and doctoral theses on signal processing and image compression under the supervision of Jens-Rainer Ohm at RWTH Aachen University in 2007 and 2012, respectively. This was followed by a brief collaboration with Javier Portilla at CSIC in Madrid, Spain, and a postdoctoral fellowship at New York University’s Center for Neural Science with Eero P. Simoncelli, studying the relationship between perception and image statistics. While there, Johannes pioneered the use of variational Bayesian models and deep learning techniques for end-to-end optimized image compression. They joined Google in early 2017 to continue working in this line of research. Johannes has served as a reviewer for publications in both machine learning and image processing, such as NeurIPS, ICLR, ICML, Picture Coding Symposium, and several IEEE Transactions journals. They have been active as a co-organizer of the annual Challenge on Learned Image Compression (CLIC) since 2018, and on the program committee of the Data Compression Conference since 2022.