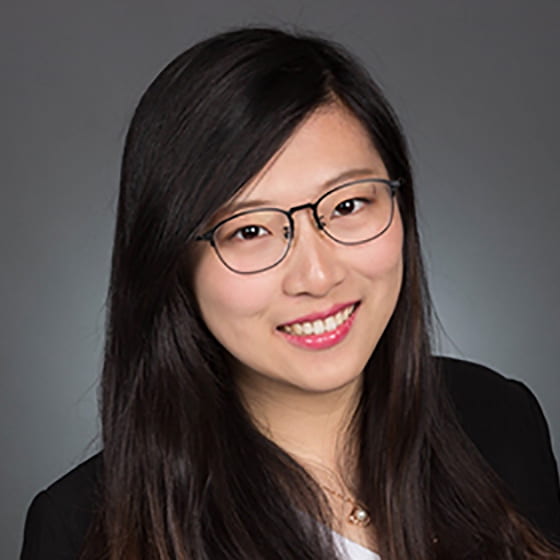
Abstract: Infinite-horizon stochastic games provide a versatile framework for studying the repeated interaction among multiple strategic agents in dynamic environments. However, computing equilibria in such games is highly complex, and the long-run outcomes of decentralized learning algorithms in multi-agent settings remain poorly understood. The first part of this talk introduces a multi-agent reinforcement learning dynamics tailored for independent and decentralized settings, where players lack knowledge of the game model and cannot coordinate. The proposed dynamics guarantee convergence to a stationary Nash equilibrium in Markov potential games, demonstrating the effectiveness of simple learning dynamics even with limited information. In the second part of the talk, we extend the learning framework to encompass Markov near potential games, offering flexibility to incorporate a wide range of practically-relevant multi-agent interaction settings. We present efficient algorithms for approximating the stationary Nash equilibrium and substantiate their effectiveness through regret analysis and numerical experiments.
Bio: Manxi Wu is an Assistant Professor at Cornell University’s School of Operations Research and Information Engineering. Her research focuses on game theory, information and market design with applications in societal-scale systems. Manxi holds a Ph.D. in Social and Engineering Systems from MIT (2021). Prior to joining Cornell, she was a research fellow at the Simons Institute for the theory of computing and the EECS department at the University of California, Berkeley (2021-2022).